Reducing the Cost of Neural Network Potential Generation for Reactive Molecular Systems
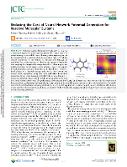
Publication date
2023Published in
Journal of Chemical Theory and ComputationVolume / Issue
19 (19)ISBN / ISSN
ISSN: 1549-9618Metadata
Show full item recordCollections
This publication has a published version with DOI 10.1021/acs.jctc.3c00391
Abstract
Although machine learning potentials have recently had a substantial impact on molecular simulations, the construction of a robust training set can still become a limiting factor, especially due to the requirement of a reference ab initio simulation that covers all the relevant geometries of the system. Recognizing that this can be prohibitive for certain systems, we develop the method of transition tube sampling that mitigates the computational cost of training set and model generation. In this approach, we generate classical or quantum thermal geometries around a transition path describing a conformational change or a chemical reaction using only a sparse set of local normal mode expansions along this path and select from these geometries by an active learning protocol. This yields a training set with geometries that characterize the whole transition without the need for a costly reference trajectory. The performance of the method is evaluated on different molecular systems with the complexity of the potential energy landscape increasing from a single minimum to a double proton-transfer reaction with high barriers. Our results show that the method leads to training sets that give rise to models applicable in classical and path integral simulations alike that are on par with those based directly on ab initio calculations while providing the computational speedup we have come to expect from machine learning potentials.
Keywords
generalized gradient approximation, dynamics simulations, exchange, water
Permanent link
https://hdl.handle.net/20.500.14178/2352License
Full text of this result is licensed under: Creative Commons Uveďte původ 4.0 International